The year 1956 is widely recognized as the inaugural year of artificial intelligence. In that year, a far-reaching symposium was held in the tranquil Dartmouth College in the town of Hanover, USA. During this symposium, participants discussed numerous issues that had not been resolved by the computer technology of the time. In this brainstorming-style meeting, the concept of "artificial intelligence" was first proposed, and artificial intelligence was officially regarded as an independent field of research.
However, due to the limitations of computer processing power at the time, artificial intelligence (AI) never made it to the forefront of practical applications. With the development of Moore's Law, the integration of chips has become increasingly dense, and computing power has seen unprecedented growth. Looking back on the development of artificial intelligence, a notable characteristic is the joint progress of computing power and algorithms. Thanks to the development of semiconductor manufacturing technology, the realization of AI has become possible.
Advertisement
With the recent surge in popularity of ChatGPT, AI has quickly gained widespread attention, sparking significant interest in the industry and stimulating the market demand for AI chips in the semiconductor industry. The world has ushered in a wave of technology led by artificial intelligence, and as a result, AI has been humorously referred to as the "fourth technological revolution."
In fact, beyond the current hype around ChatGPT and its applications in text and image production, AI is also empowering various industries. For instance, the semiconductor manufacturing field is gradually introducing AI technology.
01
EDA Tools and Artificial Intelligence
Cadence Vice President and General Manager of China, Xiaoyu Wang, believes that "Moore's Law drives process improvements, and the reduction of line widths inevitably leads to more complex and larger-scale designs. Although for economic reasons, 3DIC and advanced packaging designs can be adopted, they pose a series of challenges in terms of heat dissipation, signal integrity, electromagnetic effects, yield, and reliability. The traditional EDA design process is already struggling to meet these challenges."
Wang points out that EDA tools need to respond more quickly to new demands and require further intelligence to achieve multi-computing and multi-engine capabilities, which can accelerate the chip iteration speed and support the semiconductor industry's development into the post-Moore era. By integrating generative AI into the design process using Large Language Models (LLM) technology, verification and debugging efficiency can be effectively improved, accelerating the code iteration convergence from IP to subsystem and then to the SoC level.
As a result, Cadence has launched the JedAI platform. Through the JedAI platform, the design process can learn autonomously from a vast amount of data, continuously optimize, and ultimately reduce the manual decision-making time of designers, greatly enhancing productivity, and thus constantly improving productivity.Through the JedAI platform, Cadence will unify the big data analytics of its various AI platforms—this includes Verisium verification, Cerebrus implementation, and Optimality system optimization, as well as other third-party silicon lifecycle management systems. Utilizing the JedAI platform, users can easily manage the increasing design complexity of emerging consumer, hyperscale computing, 5G communications, automotive electronics, and mobile-related applications. Customers can deploy all their big data analytics tasks through the JedAI platform while using Cadence's analog/digital/PCB implementation, verification, and analysis software (even third-party applications).
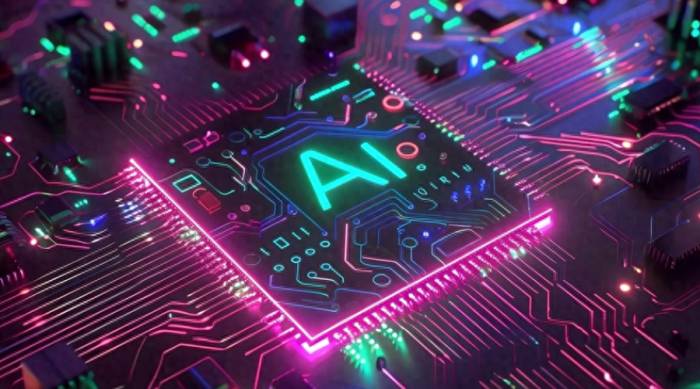
In addition, Cadence's place-and-route tool Innovus also has built-in AI algorithms to enhance the efficiency and quality of Floorplan. Project Virtus addresses the interplay between EM-IR and Timing through machine learning; tools such as Signoff Timing and SmartLEC also incorporate artificial intelligence algorithms.
Beyond Cadence, Synopsys also launched the industry's first autonomous artificial intelligence application for chip design in 2020—DSO.ai (Design Space Optimization AI). As an AI and inference engine, DSO.ai searches for optimization objectives within the vast solution space of chip design. This solution significantly expands the exploration of options in the chip design process, autonomously executing minor decisions, assisting chip design teams to operate at an expert level, and greatly improving overall productivity, thus sparking a new revolution in the field of chip design.
Combining AI technology with EDA tools offers two core values. First, it aims to make EDA smarter, reducing repetitive and complex tasks, allowing users to design chips with better PPA (Power, Performance, and Area) in the same or even less time. Second, it significantly lowers the barrier to entry for users, addressing the challenge of talent shortages.
02
OPC and Artificial Intelligence
In addition to the extensive use of AI technology in the design phase of EDA, the chip manufacturing process is also gradually incorporating artificial intelligence technology. In the semiconductor manufacturing industry, AI, especially machine learning, has comprehensive application scenarios, such as equipment monitoring, process optimization, process control, device modeling, photomask data correction, layout verification, and so on.
With the continuous miniaturization of integrated circuit devices brought by Moore's Law, there is a need to create smaller patterns on the wafer, which poses a significant challenge to wafer patterning. Photolithography technology is the main method for wafer patterning. However, with the advancement of process technology, as early as the 180-nanometer technology node, the optical image resolution of photolithography machines could no longer keep up with the development of the process due to the increasing severity of optical image distortion. To compensate for optical image distortion, the industry introduced Optical Proximity Correction (OPC) technology to compensate for optical distortion effects.
There are mainly two methods to implement OPC: Rule-Based OPC and Model-Based OPC. The early Rule-Based OPC was widely used due to its simplicity and fast computation. However, this method requires manual formulation of OPC rules, and as optical distortion becomes more severe, these rules become extremely complex and difficult to sustain. At this point, Model-Based OPC emerged. Traditional Model-Based OPC requires accurate lithography modeling, which generally includes two parts: optical modeling and photoresist modeling. Through the photoresist model, the optical image can be transformed into a photoresist pattern, and the photoresist model directly determines the accuracy of the model.
Over the past decade, advancements in computer technology have made deep learning shine. Convolutional Neural Networks (CNN) have been widely used in image processing, and OPC researchers have also applied this technology to lithography modeling. As the latest research results in artificial intelligence continue to be applied in the OPC field, from two-layer neural networks to transfer learning and even GANs, the OPC field has become a testing ground for AI applications.Defect Detection and Artificial Intelligence
As Moore's Law progresses, the chip manufacturing process becomes increasingly complex. The smaller the size of the chip circuit units, the more likely various defects are to occur during production. It is necessary to detect defects early in the production process, promptly eliminate the causes of defects, and discard defective samples to prevent defective grains from continuing processing, which would affect yield and productivity.
With the continuous reduction of linewidths, once harmless tiny particles have become defects affecting yield, making the difficulty of detection and defect correction increasingly greater. Similarly, the formation of 3D transistors and multiple process technologies have also brought subtle changes, leading to a manifold increase in defects that reduce yield.
Defects in semiconductor wafers are diverse, including topographical defects, contamination, crystal defects, and so on. At the same time, the irregularity and subtlety of semiconductor wafer defects pose great difficulties for wafer defect detection.
Currently, there are two main methods for defect detection in the semiconductor industry: Automatic Optic Inspection (AOI) and Scanning Electron Microscope (SEM) detection systems.
In the field of automatic optical inspection, given the irregularity of wafer defects, the task of target detection for wafer defects after image acquisition by image sensors often cannot cover all possible defects when processed with traditional image processing algorithms. Deep learning methods (image recognition methods based on CNN) have shown high performance in image classification and target detection, which can greatly improve the recognition rate of irregular defects and enhance the overall system performance and speed.
In 2021, the renowned semiconductor equipment company AMAT launched ExtractAI, which is based on big data and artificial intelligence. It is understood that the ExtractAI technology, developed by data scientists at Applied Materials, has solved the most challenging wafer detection problem: quickly and accurately identifying defects that reduce yield from the millions of harmful signals or "noise" generated by high-end optical scanners. ExtractAI technology can connect the big data generated by the optical inspection system with the electron beam inspection system that can classify specific yield signals in real time, thereby inferring that the Enlight system has solved all wafer map signals, distinguishing defects that reduce yield from noise. ExtractAI technology can depict the characteristics of all potential defects on the wafer defect map with detection of only one-thousandth of the samples. This results in an actionable classified defect wafer map, effectively improving the speed, ramp-up, and yield of semiconductor node development. Artificial intelligence technology can adapt to and quickly identify new defects during mass production, and its performance and efficiency gradually improve with the increasing number of scanned wafers.
In terms of electron beam, KLA introduced deep learning algorithms in its eSL10 electron beam patterning wafer defect inspection system launched in 2020, applying artificial intelligence systems to it. With its advanced artificial intelligence system, eSL10 can meet the evolving detection requirements of IC manufacturers and eliminate the most critical defects affecting device performance.
In addition to wafer defect detection in the manufacturing process, AI technology is also gradually penetrating into defect detection in the packaging and testing process. In 2020, KLA launched the Kratos1190 wafer-level packaging inspection system, ICOS F160XP chip sorting and inspection system, and the next-generation ICOS T3/T7 series packaging integrated circuit (IC) component inspection and measurement systems. The new equipment adopts AI solutions to improve yield and quality and promote semiconductor packaging innovation.In summary, traditionally, the detection of defects in optical and electron beam images requires human intervention to verify the type of defect. AI systems learn and adapt, enabling rapid classification and identification of defects, reducing errors, and not slowing down production speed.
04
Process Development and Artificial Intelligence
As chips evolve from planar structures to three-dimensional structures and beyond, new devices and processes drive material innovation. The powerful capabilities of artificial intelligence in data analysis and machine learning can accelerate the development process of semiconductor processes, thereby significantly reducing R&D cycles and costs.
Currently, NVIDIA's cuLitho computational lithography library, which has been developed, has been applied by international semiconductor equipment and semiconductor manufacturing factories, accelerating the design and production development of 2-nanometer process chips; Lam Research has accelerated deep silicon etching through artificial intelligence.
In 2023, Lam Group published a study in Nature, examining the potential of using artificial intelligence (AI) in the development of chip manufacturing processes.
To manufacture each designed chip or transistor, experienced and skilled engineers must first create a specialized recipe, outlining the specific parameters and arrangements required for each process step. Building these nanoscale devices on silicon wafers requires hundreds of steps. Process steps typically include multiple instances of depositing material thin films onto silicon wafers and etching away excess material with atomic-level precision. This important phase of semiconductor development is currently carried out by human engineers, primarily using their intuition and "trial and error" methods. Since every chip design recipe is unique and there are over 100 trillion possible options to integrate, process development can be laborious, time-consuming, and costly, increasingly slowing down the time required to achieve the next technological breakthrough.
In Lam's study, machines and human participants competed to create targeted process development recipes at the lowest cost, weighing various factors related to test batches, metrology, and management expenses. The study concluded that while humans excel at solving challenging and out-of-the-box problems, a hybrid human-first, computer-second strategy can help address the tedious aspects of process development and ultimately accelerate process engineering innovation.
In the future, intelligent integrated circuit manufacturing will leverage connectivity in factories to drive automation improvements. AI systems can process massive datasets, gain deep insights into trends and potential deviations, and use this information to make decisions.
Comment