Recently, there has been good news from Tsinghua University. They have pioneered a novel AI photonic chip architecture, developing a new AI "photonic chip" called Taichi, which can achieve 160 TOPS/W of general intelligent computing, with an energy efficiency 1000 times that of the H100.
The efficient chip for training the next generation of trillion-parameter large models has been born. Currently, the relevant research paper, titled "Large-scale photonic chiplet Taichi empowers 160-TOPS/W artificial general intelligence," has been published in the authoritative scientific journal Science.
Paper link:
01
What is the achievement?
At present, there are increasing signs that LLM will not be the ultimate path to AGI.
Computers have long become the world's energy consumption giants, and with the increasing use of energy-intensive artificial intelligence, the energy demand for computers is also rising rapidly.
Taking NVIDIA's H100 as an example, its peak power consumption is 700 watts, which, calculated at a 61% annual utilization rate, is equivalent to the average power consumption of an American household (assuming 2.51 people per household). Some experts predict that after the large-scale deployment of H100, the total power consumption will be comparable to that of a large American city, and may even exceed that of some small European countries.If a chip could be invented that saves a significant amount of energy, the performance of Large Language Models (LLMs) may achieve even greater enhancements in the future.
Advertisement
Tai Chi may make General Artificial Intelligence (AGI) a reality.
According to the official website of Tsinghua University, the Tsinghua team has designed a large-scale photonic chip named "Tai Chi," based on integrated diffractive interference heterogeneous design and a universal distributed computing architecture. This architecture has the capability of tens of millions of neurons, achieving a general intelligent computing rate of 160 trillion operations per second per watt (TOPS/W).
Furthermore, the Tai Chi photonic chip has demonstrated the ability to classify 1000 categories on a chip (with an accuracy of 91.89% on the 1623-category Omniglot dataset) and to generate high-fidelity artificial intelligence content, with an efficiency increase of two orders of magnitude.
Researchers have stated that "Tai Chi" paves the way for large-scale photonic computing and advanced tasks, further exploring the flexibility and potential of photonics in modern AGI.
Science has highly commented on this research: "The team from Tsinghua has explored a distributed diffractive interference hybrid photonic computing architecture, effectively scaling up the size of optical neural networks (ONNs) to the level of millions of neurons. They have experimentally realized an ONN with 13.96 million neurons on a single chip, for complex, thousand-category classification and artificial intelligence content generation tasks. This work is a promising step towards real-world photonic computing, supporting a variety of applications in artificial intelligence."
02
What is it, and what is it used for?
The bottleneck of electronic chips
When electrons pass through transistors and other traditional integrated circuit components, they encounter resistance and generate heat. As designers continue to add various components to the chip, the heat generated by the chip naturally increases. This characteristic of electrons has even become a barrier to the performance improvement of microchips and is also the main reason for the high energy consumption of computers.The development of technology based on electrons as carriers is approaching the physical limit, with the "power wall" dilemma emerging when chip sizes are reduced to the extreme, where a bottleneck in memory access prevents the storage and computation of a large amount of information, and the performance of electronic chips improves while the cost-performance ratio decreases.
The idea of replacing electrons with photons in circuits has been around for a long time.
In the 1960s and 1970s, researchers had already begun developing photonic chips. At that time, some experts anticipated that photonic chips would miniaturize as quickly as traditional integrated circuits.
The reduction in circuit energy consumption is attributed to the properties of light. Photonic chips do not have a resistance issue because photons generated by lasers can quickly pass through an array of components such as waveguides, modulators, and reflectors. Therefore, photonic chips produce less heat and consume less energy.
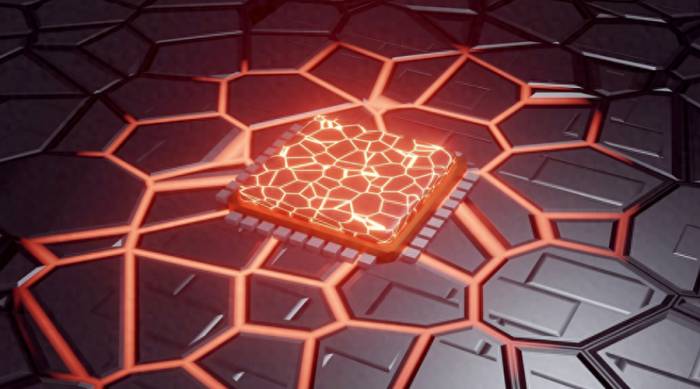
Photonic computing chips, as the name suggests, shift the carrier of computation from electricity to light, utilizing the propagation of light within the chip for computation.
The era of artificial intelligence is supported by computing power. From an energy consumption perspective, silicon photonics technology can effectively enhance the overall performance of GPUs and significantly reduce their power consumption, effectively addressing the current bottleneck in computing power. That is to say, the next generation of computing power is likely to be photonic computing or even quantum computing.
The issue lies in the fact that current integrated photonic computing, especially optical neural networks (ONNs), typically contains hundreds to thousands of parameters, with dozens of them being adjustable, only supporting basic tasks such as simple pattern recognition and vowel recognition. They can perform some simple tasks and shallow models but cannot support the complex large model intelligent computing that urgently requires high computing power and high energy efficiency.
Photonic computing demands a higher level of integration, but the technical challenges are not only in integration; there are greater challenges in the recycling of computing units and nonlinear processing.
It is understood that in a massive AI model, each layer of the network needs to perform matrix operations, and a large matrix may need to be broken down into several smaller matrices for computation. That is to say, in large models, matrix multiplication and addition operations are a repetitive and cyclical computational process, and each cycle, the element weights on the matrix are updated.Compared to electronics, light performs matrix multiplication and addition operations very quickly, but when it comes to updating weights, the speed of light slows down.
To achieve large-scale, energy-efficient photonic computing, it is unrealistic to simply scale up existing photonic neural network chips, because as the number of neural network layers increases, inevitable analog noise will expand exponentially. Scaling up the size of existing architectures does not proportionally improve performance.
New architecture: Distributed diffraction-interference hybrid photonic computing architecture
The Tsinghua team conducted architectural research, "from 0 to 1" redesigning a new architecture suitable for photonic computing.
According to the paper, the Tsinghua team built a network structure for Taichi, which adopts distributed computing, with a shallow depth but a broad width.
Different from the traditional method of stacking a series of layers for deep computing, Taichi distributes computing resources to multiple independent clusters, organizes clusters for subtasks separately, and finally synthesizes these subtasks to complete complex high-level tasks.
Specifically, the fully connected characteristics of the optical diffraction layer can provide greater deformation capabilities than the convolutional layers in traditional DNNs.
This means that optical networks have the potential to achieve the same transformation with fewer layers than electronic systems.
The first author of the paper, Dr. Xu Zhihao from the Department of Electronics, introduced: "In the 'Tai Chi' architecture, the top-down encoding splitting-decycling reconstruction mechanism simplifies complex intelligent tasks, breaking them down into multi-channel high-parallel subtasks. The distributed 'large receptive field' shallow-layer optical network divides and conquers the subtasks, breaking through the inherent computational errors of multi-layer deep cascading in physical simulation devices."AI Photonic Chip: Interference-Diffraction Hybrid Computing Chip
In this work, the team designed a large-scale diffraction-interference hybrid photonic AI chip with a flexible distributed computing architecture—known as "Tai Chi."
According to the paper: The "Tai Chi" photonic chip boasts an area efficiency of 879 T MACS/mm² and an energy efficiency of 160 TOPS/W, achieving an order-of-magnitude improvement in energy efficiency. It empowers optical computing for the first time to perform complex AI tasks such as the recognition of thousands of objects in natural scenes and cross-modal content generation.
03
What is the situation of domestic photonic chip companies?
Photonic chips, as a potential disruptive technology for the "post-Moore era," have come into view. With their high speed, low power consumption, and relatively mature process technology, they can effectively break through the bottlenecks of the physical limits of traditional integrated circuits. They meet the technological demands of artificial intelligence, the Internet of Things, cloud computing, and other industries in the new round of technological revolutions for information acquisition, transmission, computation, storage, and display.
At present, the global photonic chip industry is just beginning. As a new integration technology independent of electronic integration technology, its technical barriers have not yet formed. China's photonic industry development level is on par with the world, with certain advantages in the basic theoretical research and technological development of photonics.
Currently, high-power laser chips and some high-speed laser chips (10G, 25G, etc.) in China are in the stage of accelerated breakthrough in domestication, while photodetection chips and high-speed laser chips above 25G are just starting.
It is worth noting that Huawei also has some layout in the field of photonic chips.
In March of this year, Huawei disclosed an invention patent for "photonic chip and its preparation method, communication equipment." The application publication number is: CN117616316A, and the patent application date is September 18, 2021. The abstract shows that the embodiments of this application provide a photonic chip and its preparation method, communication equipment, involving the field of optical communication technology, to solve the problem of easy breakage at the tip of the optical waveguide during the preparation process of the existing photonic chips.Profit Decline in Optical Chip Companies
Domestic optical chip manufacturers, including Active Jie Technology, Changguang Huaxin, and Jiguang Technology, have all cited the impact of macroeconomic conditions and industry development in their 2023 annual performance reports, leading to reduced product demand and lower prices.
Active Jie Technology, a leading domestic integrated device manufacturer (IDM) of optical chips, offers products ranging from 2.5G to 50G indium phosphide laser chips. Looking at the company's 2023 performance, the total operating income was approximately 144 million yuan, a 48.96% decrease compared to the same period last year, with a net profit attributable to shareholders of 19.5 million yuan for the full year, a reduction of 80.58% year-on-year.
Changguang Huaxin's performance report also indicates a loss-making status. The operating income was about 292 million yuan, a 24.2% decrease year-on-year; the net profit attributable to shareholders of the listed company was a loss of 86.1017 million yuan. From January to June 2023, the revenue composition of Changguang Huaxin was as follows: high-power single-tube series accounted for 90.16%, high-power bar series accounted for 8.24%, other businesses accounted for 0.9%, and VCSEL chip series accounted for 0.7%.
Jiguang Technology's business covers the upstream "photon generation" and "photon control" as well as the midstream automotive, pan-semiconductor, and healthcare sectors, having established cooperation with several well-known companies in the industry. Jiguang Technology's 2023 annual performance report shows an operating income of about 561 million yuan, a 1.69% increase year-on-year; the net profit attributable to shareholders of the listed company was 89.68 million yuan, a 29.44% decrease year-on-year.
Active Jie Technology stated "sales in the telecommunications market and data centers did not meet expectations"; Changguang Huaxin mentioned "due to the impact of the macroeconomic environment and other factors, market confidence is insufficient, the demand for laser devices continues to be sluggish, and industry competition intensifies"; Jiguang Technology noted "the prices of some upstream component products of the company have decreased, leading to a decline in the overall gross margin."
Comment