Under the wave of artificial intelligence, the development of photonic chips is accelerating.
As one of the three main drivers of artificial intelligence, computing power is key to training AI models and reasoning tasks.
A new achievement by a research team from Tsinghua University was published in the latest issue of "Science" on the morning of April 12th. They pioneered a distributed wide-intelligence photonic computing architecture and developed the world's first large-scale interference diffraction heterogeneous integrated chip "Tai Chi," achieving a universal intelligent computing of 160 TOPS/W.
It is reported that during the development of the "Tai Chi" photonic chip architecture, the team was inspired by the ancient text "I Ching." The team members took the phrase "Yi has Tai Chi, which gives birth to the two instruments" as a source of inspiration, establishing a brand-new computing model that unleashes the powerful performance of photonic computing.
Photonic computing, as the name suggests, changes the carrier of computation from electricity to light, using the propagation of light within the chip for computation. With its ultra-high parallelism and speed, it is considered one of the most promising competitive solutions for future disruptive computing architectures.
Advertisement
Photonic chips, with their advantages of high-speed and high-parallel computing, are expected to support advanced artificial intelligence applications such as large models.According to the first author of the paper, Xu Zhiwu, a doctoral student in the Department of Electronics, the top-down encoding splitting-decoupling mechanism in the "Tai Chi" architecture simplifies complex intelligent tasks by breaking them down into multiple high-parallel sub-tasks. The distributed "large receptive field" shallow optical network constructed for these sub-tasks overcomes the inherent computational errors of multi-layer deep cascading in physical simulation components.
The paper reports that the "Tai Chi" optical chip has an area efficiency of 879T MACS/mm and an energy efficiency of 160 TOPS/W. For the first time, it empowers optical computing to achieve complex artificial intelligence tasks such as the recognition of thousands of object categories in natural scenes and cross-modal content generation.
The "Tai Chi" optical chip is expected to provide computational support for large model training and inference, general artificial intelligence, and autonomous intelligent unmanned systems.
Artificial intelligence requires photonic circuits.
Artificial intelligence typically relies on artificial neural networks for applications such as analyzing medical scans and generating images. In these systems, circuit components known as neurons (similar to those in the human brain) are fed with input data and collaborate to solve problems, such as recognizing faces. If the neural network has multiple layers of these neurons.
As the scale and power of neural networks grow, they become increasingly energy-consuming when running on traditional electronic devices. For example, to train its state-of-the-art neural network GPT-3, a study in the 2022 issue of the journal Nature showed that OpenAI spent $4.6 million to run 9,200 GPUs over two weeks.
The drawbacks of electronic computation have led some researchers to explore optical computing as a promising foundation for the next generation of artificial intelligence. Compared to their electronic counterparts, this photonic approach uses light to perform computations more quickly and with lower power.Tsinghua University has led the development of a photonic microchip named Taichi, which can perform advanced artificial intelligence tasks just like electronic devices, while also being proven to be more energy-efficient.
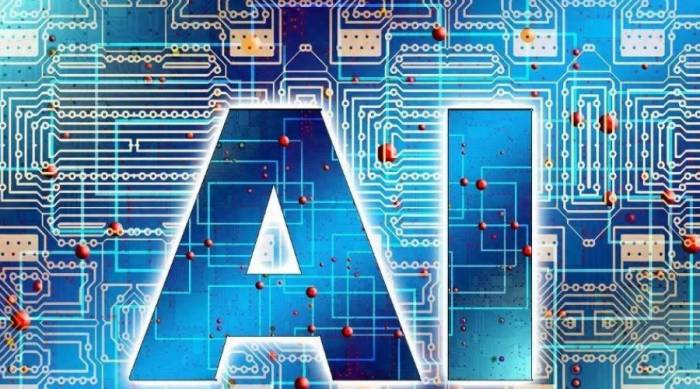
"Optical neural networks are no longer toy models," said Lu Fang, an associate professor of electronic engineering at Tsinghua University. "They can now be applied to real-world tasks."
How do optical neural networks work?
There are two main strategies for developing optical neural networks: 1) Scattering light in specific patterns within the microchip; 2) Allowing light waves to interfere with each other in a precise manner within the device. When input into these optical neural networks in the form of light, the output light encodes data for the complex operations performed within these devices.
Fang explained that both photonic computing methods have distinct advantages and disadvantages. For instance, optical neural networks that rely on scattering or diffraction can pack many neurons closely together and consume almost no energy. Diffraction-based neural networks depend on the scattering of light beams as they pass through optical layers representing the operations of the network. However, a disadvantage of diffraction-based neural networks is that they cannot be reconfigured. Each operation string is essentially only usable for a specific task.
In contrast, interference-based optical neural networks can be easily reconfigured. Interference-based neural networks send multiple beams through a grid of channels, and the way they interfere at the intersections of these channels helps perform the operations of the device. However, their downside is that interferometers are also bulky, which limits the scalability of such neural networks. They also consume a significant amount of energy.
Furthermore, current photonic chips encounter inevitable errors. Attempting to scale up optical neural networks by increasing the number of neuron layers in these devices typically only multiplies the noise. This means that, up to now, optical neural networks have been limited to basic artificial intelligence tasks, such as simple pattern recognition, in other words, optical neural networks are generally not suitable for advanced applications.
Researchers say that, in contrast, Taichi is a hybrid design that combines diffraction and interference methods. It includes clusters of diffraction units that can compress data for large-scale input and output in a compact space. The chip also includes an array of interferometers for reconfigurable computing. Fang stated that the encoding protocol developed for Taichi will divide challenging tasks and large network models into sub-models that can be distributed across different modules.How does Taichi integrate these two types of neural networks?
Previous research often tried to expand the capacity of optical neural networks by imitating what their electronic counterparts frequently do—adding more layers of neurons. Taichi's architecture expands by distributing computations across multiple small chips that run in parallel, which means Taichi can avoid the exponential accumulation of errors that occur when optical neural networks stack many layers of neurons together.
"This 'shallow depth, wide width' architecture ensures the scale of the network," says Fang.
*Disclaimer: This article is the original creation of the author. The content of the article represents their personal views, and our reposting is solely for sharing and discussion, and does not represent our approval or agreement. If you have any objections, please contact the backend.
Comment